Cautious learning
Manuscript in preparation
Zago, D., Capizzi, G. (2023). Alternative parameter learning schemes for monitoring process stability. Quality Engineering. https://doi.org/10.1080/08982112.2023.2253891
Description
Control chart statistics $C_{t}$ that are based on estimated model parameters are usually applied with one of the following approaches:
- Fixed-parameter (FE): the model parameters are held fixed after estimation on an in-control dataset.
- Adaptive estimator (AE): the model parameters are recursively updated after having checked for potential OC situations.
In this work, we formally show how these approaches are opposite examples of bias-variance trade-off, and as such they suffer from complementary issues. With fixed estimators, large amounts of data are required to be effective in detecting shifts. With adaptive estimators, early and small shifts go undetected from the control chart and OC observations bias the estimate, thereby reducing performance.
Cautious learning
We propose an improvement over both approaches by choosing a “middle ground”, namely an estimator that alternates between the fixed-parameter and adaptive estimator approaches. We term this approach cautious learning (CL) in order to underline how the parameter updates are stopped to prevent biasing the estimates,
\[\widehat{\theta}_{t} = \begin{cases} \widehat{\theta}(\mathbf{y}_{1:t}) & \text{if $C_{t} > 0$}\\ \widehat{\theta}_{t-1} & \text{if $C_{t} = 0$}\\ \end{cases}\]Combining the proposed approach with the GICP methodology for designing control limits results in a considerably higher detection power for early and small shifts, while maintaining similar performance to the adaptive estimator for large and delayed shifts.
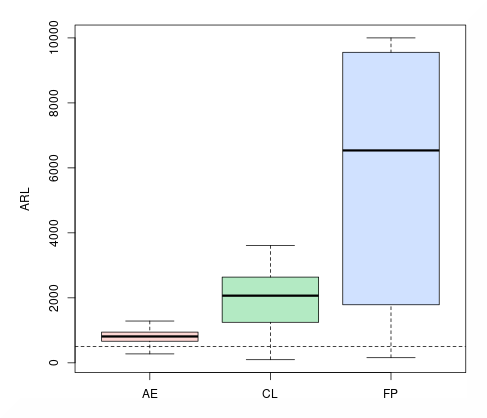
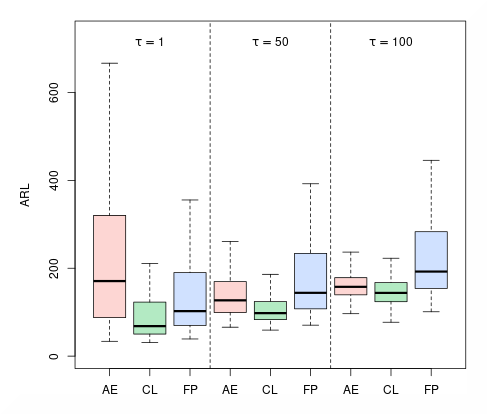